Forecasting sales using previous sales data
Sales forecasting methods are very varied and can incorporate a multitude of internal and external data. Among these methods, we find the one that consists in using historical data to anticipate future sales.This is a widely used solution in companies, as it is relatively easy to set up, either by creating a forecast file on Excel or by using a sales forecasting software. Nevertheless, this method suffers from some drawbacks, and it should not be considered exclusively, at the risk of not producing sufficiently reliable forecasts.
That's why we take a look at how historical data works, the advantages of the method and its limitations, so that you can use it wisely!
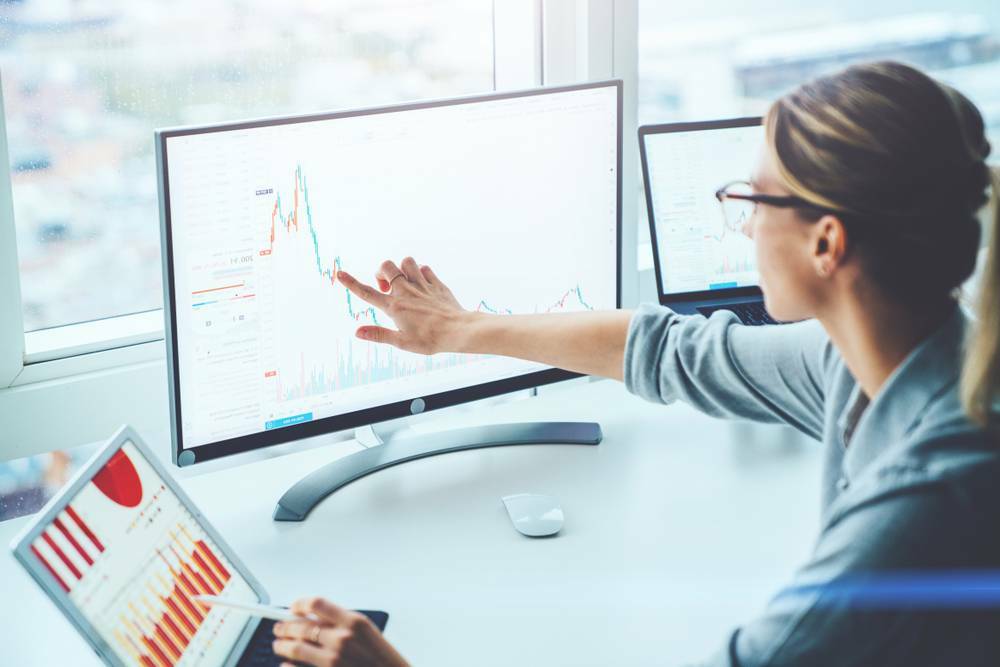
What is the historical data method?
The principle of the method is actually quite simple: use sales data from the last few months, quarters or even years to predict how much product will be sold in the coming months. In many cases, this involves estimating that sales will be equal to or greater than in past periods, based on the growth rate over the previous period studied.The use of historical data is therefore a comparison method, which works with internally collected information. In other words, it only concerns the company's sales, but does not include figures from competitors, market developments, etc.
Within this generic method, several models can be used to determine the forecast:
- simple linear regression, to establish a formula to calculate the number of products sold for the next period;
- adjustment using the extreme points method, which allows to draw a straight line between two points and gives the trend of sales evolution;
- adjustment using the Mayer method, which requires the calculation of two average points instead of the extreme points;
- definition of the least squares line, drawn according to a formula similar to the two previous adjustments, but including additional calculations to obtain adjusted and more reliable values;
- calculation of the moving average, simple or weighted, which consists in making successive averages to predict future sales;
- exponential smoothing, which may or may not take into account trend and seasonality;
- imputation of a seasonal coefficient for the forecast calculation, in order to predict sales according to periods of greater or lesser activity.
These are the main quantitative methods based on historical data: some are very simple to implement, while others require a little more knowledge of statistics and probability. But with today's tools (CRM software, dedicated sales forecasting solution, pre-built Excel formulas, etc.), all these calculations can be automated. The sales, finance and marketing teams then have at their disposal tables and graphs that offer them a simplified overview of expected sales.
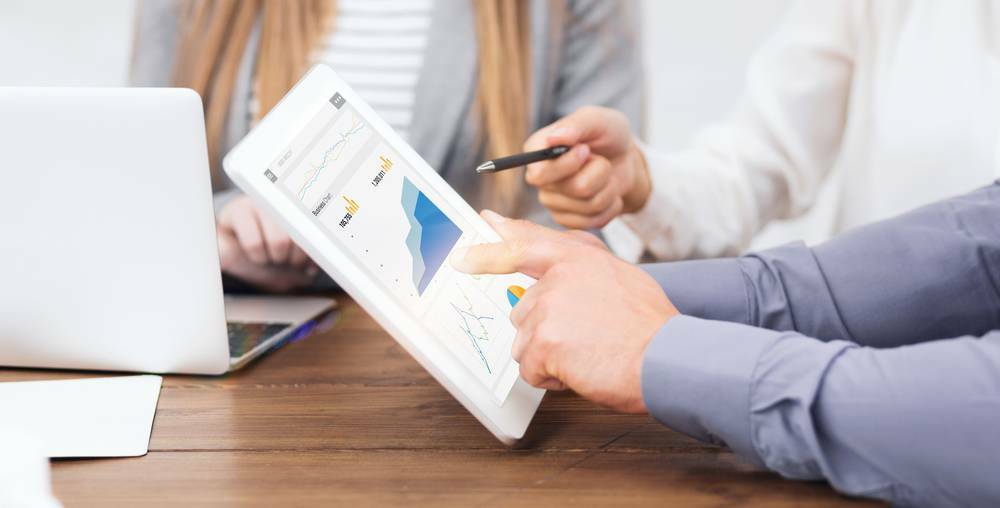
Why promote this method of prediction?
Forecasts based on historical data have the major advantage of being simple to implement. The methods and formulas used have been known for a long time and are taught in marketing, logistics and sales management courses. By using this method, everyone in the company who needs to make, analyze and read sales forecast results is able to do so. We are not dealing with a technique reserved only for experts, who would make calculations on their own in a way that is opaque and difficult to understand by the other employees of the company.Moreover, this method allows you to quickly create a forecast plan, especially when using an appropriate CRM software. The sales data are automatically integrated and it is then sufficient to generate a forecast according to the desired criteria and variables, to obtain a short or medium term vision of the sales over a desired period.
The other strong point of the historical forecast is the use of concrete and reliable data. Even if it is impossible to predict the future with certainty, it is undeniable that the figures reflect the economic and commercial reality of the company. By relying on information that cannot be disputed, it is easier to determine coherent objectives and have them accepted by the sales and marketing teams.
What are the limitations of using historical data?
The simplicity of the historical forecasting model is what makes it successful, but it is also what limits its possibilities and calls into question its level of accuracy.Indeed, with such a method centered on probability and statistics, it is essential to have a large quantity of data, over a sufficiently long period, in order to obtain satisfactory results. However, a company that is only in its first year of activity does not have this essential data. Therefore, it has no choice but to use market research, its own resources and external data to estimate the number of sales.
But the central problem with this type of forecasting, especially when using a very simplified calculation model, is that it does not sufficiently take into account contextual elements. For example, the calculation of an average does not allow for the detection of the seasonality of sales, which has a major impact on the distribution of revenues over the year. And even if you apply a coefficient or use Winters' exponential smoothing model, you will need to have data on several sales cycles to make a reliable forecast.
Beyond the problem of seasonality, it is important to be aware that forecasting based on historical sales data is not intended to determine a long-term plan. Indeed, by using only past information, we do not take into account all the context that can affect sales (state of competition, evolution of consumer needs, launch of a new product, etc.).
These limitations should lead to the conclusion that the historical forecasting method is a useful tool, but it is not sufficient to conduct a thorough analysis and an effective business strategy. The forecasts that emanate from it can be used as a basis for determining the production plan, allocating resources and setting objectives, but they are only one component of a richer forecast. For example, it is appropriate to supplement these forecasts with other methods, taking into consideration the sales cycle of a product, consumer surveys, industry trends, etc.
We recommend these other pages: