How to calculate forecasted sales?
To optimize business processes and ensure profitability, sales forecasting is an indispensable part of any company's business plan. If sales volumes and the resulting revenue potential are incorrectly forecast, the company's performance can be affected. That is why the sales team responsible for forecasting must do everything in its power to continuously improve its sales forecasts.To do this, they must make precise calculations. Among all the methods of calculating sales forecasts, the company will have to choose the most relevant ones, especially according to the data it has.
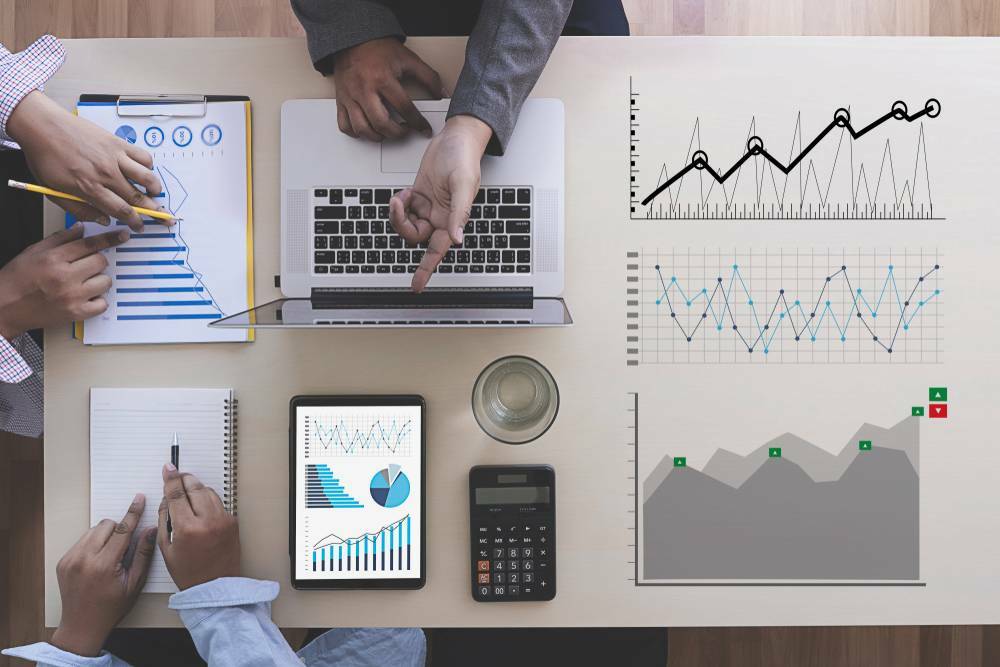
Calculate sales forecasts with simple quantitative methods
The basic principle of simple quantitative methods
Simple quantitative methods are based on the use of mathematical formulas obtained from a graphical representation or a summary table of past data. The graphical representation takes the form of a more or less straight cloud of points, each point symbolizing a piece of data. For sales forecasts, the graph generally illustrates the evolution of the sales volume or turnover according to years, months or quarters.The three methods of linear forecasting, or linear adjustment, consist in determining and drawing a line that passes as close as possible to each point of the scatter plot. From the analysis of this line, a trend can be identified. Assuming that sales evolve in a linear fashion, forecasts for future periods can be made by extrapolating the data.
The extreme points method
The method of extreme points, the simplest to use, can be applied when the variables considered evolve in a very regular and linear way. This method consists in determining the equation of the line of type y = ax + b, where:
- y is the sales volume (or turnover);
- x is the year sought for the forecast;
- a and b are parameters independent of x, with a the directing coefficient of the line and b a constant.
To determine the forecasted revenue or sales volume, simply replace x with the year of interest. For example, if we consider the period from 2010 to 2015, and we want to forecast the year 2016, we replace x by 6 since this is the sixth year since 2010. We then get y, the expected sales volume or revenue for the year 2016.
The double mean method
The double mean method, or Mayer's method, can be applied when the variables considered evolve in a fairly regular and linear way. In the same way as for the extreme points method, it consists in solving the equation of the linear adjustment line.However, this second technique for calculating forecasted sales requires the division of the data series into two groups. To solve the system of equations, the coordinates of the mean point of each group are used.
In this way, Mayer's method takes into account all the data in the series, thus ensuring more accurate forecasts than those obtained by the extreme point method.
The least squares method
Although relatively complex at first glance, the least squares method is the most accurate linear fitting method and the most suitable for more dispersed data, i.e. when the evolution of the company's activity is not stable.Like the other methods, the principle is to solve the equation y = ax + b. However, the least squares method incorporates the calculation of averages to determine the equation of the line:
- y is the sales volume (or turnover), from which the average of the volumes (or turnover) is subtracted;
- x is the year sought for the forecast, from which the average of the years is subtracted;
- a is the sum of x x y, which is divided by the sum of x²;
- b is determined by subtracting the value of a multiplied by the average of the years from the average of the volumes (or sales).
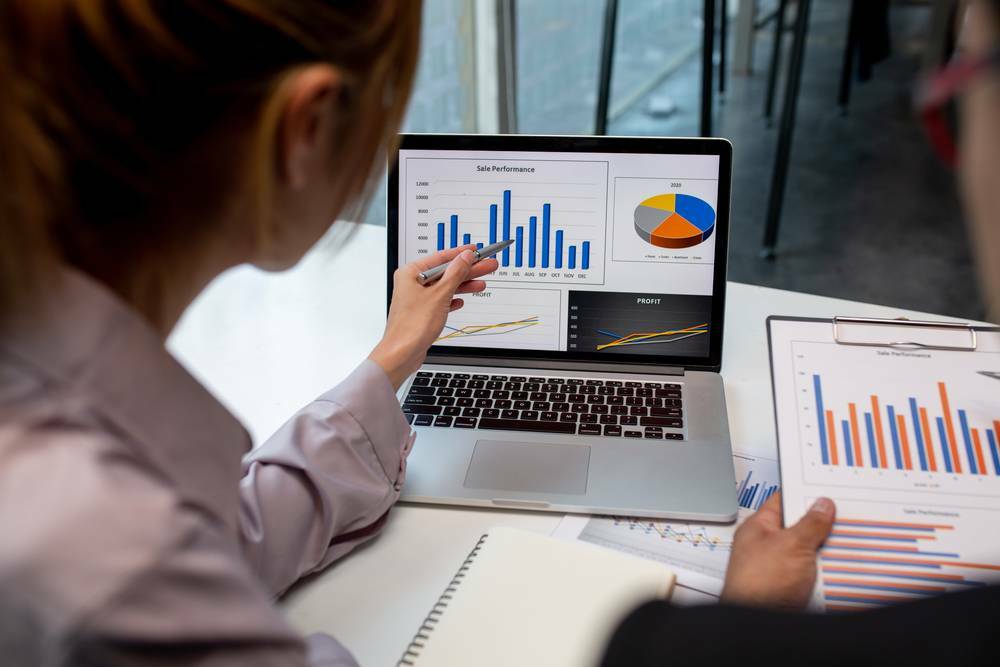
Calculate forecasts with other quantitative methods
The regression and correlation method
To perform a linear regression, the company will use the least squares method. The simple regression method consists in highlighting the relationship between two variables, one dependent on the other.Multiple regression is used to analyze the relationship between a dependent variable (revenue or sales volume) and several other independent variables. Given the accuracy of the calculation, this is one of the most common methods used by companies wishing to forecast the sales of a product or several products.
To better appreciate the quality of the forecasting using the regression method, the correlation coefficient must be measured. The correlation expresses the intensity of the link between the variables studied. The formula for the correlation coefficient, which is rather complex at first glance, relates the variables under study to their respective averages. The coefficient is between 1 and -1: when it is close to one of the two extremes, the sales forecast is all the more precise and reliable.
The exponential smoothing method
Exponential smoothing is not the most commonly used method of calculating sales forecasts. Like other quantitative methods, the smoothing technique is based on the same principles of extrapolation of past data. However, exponential smoothing gives more weight to the most recent data, thanks to the calculation of a weighting coefficient. This method can be used by a company wishing to forecast its sales volume on a global market.
The moving average method
In order to calculate the moving average of a time series, the sales or turnover averages for each period (year, quarter or month) must first be available. Then, the period considered must be divided into several periods (a few months or a quarter). The average for each period is calculated based on the averages of the previous periods.From the moving averages obtained, it is possible to highlight the seasonality of the data series by calculating seasonal coefficients. The calculation of these seasonal coefficients is more interesting when the company wants to forecast the monthly sales of its products or services.
While some sales forecasting calculations are relatively simple to perform, others require more skill on the part of the forecasting team. However, there is no need to worry: today's management tools such as predictive models can automate all these calculations.
We recommend these other pages: